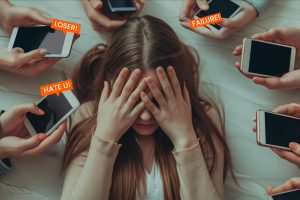
Knorex R&D Team Publishes Research on Advancing Hate Speech Detection Technology
We are thrilled to announce the publication of our R&D team’s latest research paper, “Disentangling Hate Across Target Identities,” which is now available on arXiv,
We build AI-driven advertising technologies and solutions for marketers.
Knorex R&D members come from these world-leading universities, institutes, and organizations in AI and NLP.
We have intern and research stay positions available all year round. Please email hr@knorex.com to apply.
We are thrilled to announce the publication of our R&D team’s latest research paper, “Disentangling Hate Across Target Identities,” which is now available on arXiv,
We’re delighted to share that Knorex R&D team’s research paper titled “Towards Weakly-Supervised Hate Speech Classification Across Datasets” was accepted to The 7th Workshop on Online
Knorex’s research scientist, Vishakha Kadam will be presenting the research paper titled “Plot Writing From Pre-Trained Language Models” and “Automated Ad Creative Generation” at the
Jin, Y., Wanner, L., & Koya, A.M. (2024). Disentangling Hate Across Target Identities. arXiv preprint arXiv:2410.10332.
Huang, C., Jin, Y., Ilievski, I., Lei, W., & Lv, J. (2024). ARAIDA: Analogical Reasoning-Augmented Interactive Data Annotation. In Proceedings of the 62nd Annual Meeting of the Association for Computational Linguistics (ACL 2024), pages 10660–10675, Bangkok, Thailand.
Jin, Y., Wanner, L., & Shvets, A. (2024). GPT-HateCheck: Can LLMs Write Better Functional Tests for Hate Speech Detection?. In Proceedings of the 2024 Joint International Conference on Computational Linguistics, Language Resources and Evaluation (LREC-COLING 2024). Turin, Italy.
Jin, Y., Wanner, L., Kadam, V., & Shvet, A. (2023). Towards Weakly-Supervised Hate Speech Classification Across Datasets. Proceedings of the 7th Workshop on Online Abuse and Harms (WOAH 2023). Toronto, Canada.
Kadam, V., Jin, Y., & Nguyen-Hoang, B. D. (2022). Automated Ad Creative Generation. Proceedings of the 15th International Conference on Natural Language Generation (INLG). Maine, USA.
Jin, Y., Kadam, V., & Wanvarie, D. (2022). Plot Writing From Pre-Trained Language Models. Proceedings of the 15th International Conference on Natural Language Generation (INLG). Maine, USA.
Jin, Y., Knorex Pte Ltd, 2021. Cross-domain contextual targeting without any in-domain labelled data. U.S. Patent 11,093,969.
Jin, Y., Bhatia, A., & Wanvarie, D. (2021, June). Seed Word Selection for Weakly-Supervised Text Classification with Unsupervised Error Estimation. In Proceedings of the 2021 Conference of the North American Chapter of the Association for Computational Linguistics: Student Research Workshop (pp. 112-118).
Jin, Y., Kadam, V., & Wanvarie, D. (2021, June). Bootstrapping Large-Scale Fine-Grained Contextual Advertising Classifier from Wikipedia. In Proceedings of the Fifteenth Workshop on Graph-Based Methods for Natural Language Processing (TextGraphs-15) (pp. 1-9).
Jin, Y., Bhatia, A., Wanvarie, D., & Le, P. T. (In press). Towards Improving Coherence and Diversity of Slogan Generation. Natural Language Engineering, 1, 33.
Jin, Y., Wanvarie, D., & Le, P. T. (2022). Learning from noisy out-of-domain corpus using dataless classification. Natural Language Engineering, 28(1), 39-69.
Charoenphakdee, N., Lee, J., Jin, Y., Wanvarie, D., & Sugiyama, M. (2019, November). Learning Only from Relevant Keywords and Unlabeled Documents. In Proceedings of the 2019 Conference on Empirical Methods in Natural Language Processing and the 9th International Joint Conference on Natural Language Processing (EMNLP-IJCNLP) (pp. 3993-4002).
Jin, Y., Wanvarie, D., & Le, P. T. (2019, April). Bridging the Gap Between Research and Production with CODE. In Pacific-Asia Conference on Knowledge Discovery and Data Mining (pp. 277-288). Springer, Cham.
Nguyen-Hoang, B. D., Pham-Hong, B. T., Jin, Y., & Le, P. T. (2018). Genre-oriented web content extraction with deep convolutional neural networks and statistical methods. In Proceedings of the 32nd Pacific Asia Conference on Language, Information and Computation.
Jin, Y., Wanvarie, D., & Le, P. (2017, November). Combining lightly-supervised text classification models for accurate contextual advertising. In Proceedings of the Eighth International Joint Conference on Natural Language Processing (Volume 1: Long Papers) (pp. 545-554).
Jin, Y., Wanvarie, D., & Le, P. (2017, November). Combining lightly-supervised text classification models for accurate contextual advertising. In Proceedings of the Eighth International Joint Conference on Natural Language Processing (Volume 1: Long Papers) (pp. 545-554).
Jin, Y., & Le, P. (2016). Selecting domain-specific concepts for question generation with lightly-supervised methods. In Proceedings of the 9th International Natural Language Generation Conference (pp. 133-142).
XPO Platform
Resources
Company
Data, Privacy & Protection
Newsletters & Updates
By subscribing, you have read and understood our Terms of Use and Privacy Policy, and you agree to receive marketing communications of our products and services. You may opt out anytime.